The known: Electronic decision support tools in general practice, combination therapy with evidence‐based cardiovascular medications (polypills), and pharmacy programs that promote medication adherence each improve cardiovascular disease (CVD) risk factor management.
The new: Uptake by participating general practices and pharmacies of three evidence‐based strategies for improving CVD risk factor management was disappointing, and the intervention had no significant impact on the management of blood pressure and LDL cholesterol in undertreated patients at high risk of CVD.
The implications: Implementing complex quality improvement initiatives is difficult. Process evaluation will provide further information about factors that hindered more widespread adoption of the intervention.
Cardiovascular disease (CVD) remains a major health challenge in Australia and around the world.1,2 Despite the widespread availability of effective and affordable preventive therapies (blood pressure‐lowering drugs,3 statins,4 antiplatelet medications5), gaps between evidence and practice persist, and in Australia they have not narrowed over the past decade.6,7
Strategies for closing these gaps in general practice have shown promise, including electronic decision support8 and fixed dose combinations of preventive drugs.9 The Treatment of cardiovascular Risk in Primary care using Electronic Decision support (TORPEDO) study,10 an Australian trial of the HealthTracker computerised decision support application, improved CVD risk factor screening by 25% (compared with usual care), and increased preventive drug prescribing for undertreated patients at high CVD risk by about 60%.
The Single Pill to Avert Cardiovascular Events (SPACE) collaboration undertook a prospective meta‐analysis of randomised trials that compared the use of polypills (containing aspirin, blood pressure‐lowering drugs, and a statin) with usual care,9 including the Australian Kanyini–GAP study.11 The polypill‐based strategy was associated with greater use of indicated treatments and lower systolic blood pressure and low‐density lipoprotein (LDL) cholesterol levels at 12 months; among undertreated people with high CVD risk, it was associated with a four‐fold increase in the use of indicated medications.9
These studies, however, were conducted in highly motivated general practices, making the generalisability of their results unclear. Further, process evaluations identified a number of limitations, including technology challenges in decision support and limited prescriber flexibility with respect to the number of polypills available.12 The polypill trials focused on efficacy and did not evaluate implementation strategies.
In the Integrated combination Therapy, Electronic General practice support tool, pharmacy‐led intervention and combination Therapy Evaluation (INTEGRATE) study, we evaluated a multifaceted intervention comprising an updated version of HealthTracker, with enhanced central support and new algorithms to identify patients who could benefit from using polypills, a broader range of polypills, and a pharmacy‐based adherence support program for promoting medication adherence.13 We evaluated the impact of this intervention on CVD risk factor control, compared with usual care, in Australian general practices.
Methods
Study design and participants
INTEGRATE was a parallel arm, cluster randomised trial in 71 community‐based general practices undertaken during 5 December 2016 – 13 September 2019. The protocol has been published,14 and the trial was registered with the Australian New Zealand Clinical Trials Registry (ACTRN12616000233426; 19 February 2016). Practices were eligible for participation if they predominantly used an electronic medical record (EMR) system compatible with HealthTracker and were willing to implement all components of the INTEGRATE intervention. Practices were recruited through established networks of the members of the study steering committee, through advertising by Primary Health Networks, and by direct approaches to general practices. Practices were randomised centrally by computer‐assisted block permutation (1:1), stratified by practice size (less than 500 or 500 or more patients in the baseline data extract), to the intervention or usual care groups. Health care providers, patients, and the study team were not blinded to allocation.
Procedures: intervention group
We used the behaviour change wheel model15 and the findings of process evaluations of specific intervention components12,16 to hypothesise how the intervention components might interact and contribute to behaviour change.14 Prior to randomisation, a pilot study was conducted in three practice–pharmacy pairs over six months to finalise the intervention (Supporting Information, 1. Pilot testing of the intervention).
At set‐up, in‐person training was provided at intervention practices by a project officer and a medically trained investigator. HealthTracker was installed on all intervention practice computers and clinic staff were trained in its use. Opening a patient EMR file triggered the presentation of immediate, tailored, guidelines‐based17,18,19 recommendations according to individual CVD risk, including screening tests required and medication recommendations (including suitability for a polypill), and an interactive risk communication tool (Supporting Information, figure 1). A population health audit tool allowed practices to identify performance deficits and to use patient recall systems (Supporting Information, figure 2). Quarterly benchmarking reports allowed practices to view peer‐ranked performance (Supporting Information, figure 3).
A tiered academic detailing system (education program) supplemented emailed benchmarking reports. Every three months, practices with scores in the lowest third of performance reports were visited by a clinically trained investigator; those in the middle third were contacted by telephone. The visits, phone calls, and emails each provided tailored advice about improving implementation of the intervention. Additionally, each intervention practice was visited at least once by consultants (Train IT Medical) specialised in training general practices in optimal EMR use, performance auditing, and change management strategies.
Eight different polypills (containing two half‐dose blood pressure‐lowering drugs and a statin, with or without aspirin) were made available by prescription at partner pharmacies. As cost may affect adherence, pharmacies charged each patient the equivalent of a single co‐payment, as defined by the Pharmaceutical Benefits Scheme (Supporting Information, table 1).
Partner pharmacies used a mobile tablet‐based decision support tool to offer the Pharmacy Adherence Support Service (PASS) to patients prescribed any preventive CVD medication (including polypills) by trial doctors. The Brief Medication Questionnaire‐1 (BMQ‐1)20 was administered to patients who scored higher than 7 on the Adherence to Refills and Medications Scale (ARMS‐7)21 to identify barriers to medication adherence and generate tailored intervention recommendations (Supporting Information, 2. Pharmacy Adherence Support Service). Adherence and modified interventions were assessed at follow‐up visits at one, six and 12 months as needed.
Participating pharmacies were supported by videos about how to use PASS. Project officers supported the use of PASS in pharmacies, alternating between telephone calls and in‐person visits every three months.
Procedures: control group
No intervention components were implemented at the control practices, but they received 6‐monthly study newsletters and brief emails or phone calls to facilitate the EMR data extraction required.
Payments
All general practices were paid a participation fee of $500, and doctors were eligible for continuing professional development (CPD) points. Pharmacies were offered initial $500 payments, in addition to $500 after completion of training and $50 per patient who participated in PASS. Pharmacists retained polypill co‐payments, as is usual practice.
Data collection
Data for all adult patients who had visited a participating practice at least three times during the preceding two years and at least once during the past six months (Royal Australian College of General Practice definition of an “active patient”22) were included. At baseline and at study end, data were collected using the CAT 4 automated data extraction tool (PEN Computing Systems) (Supporting Information, figure 4). A de‐identified data subset from each practice EMR system was extracted, including demographic information, selected medical history, medications, and CVD risk factor assessments. Each dataset contained individual patient identifiers to allow record matching of baseline and follow‐up data; to be included in any evaluation, patients’ data were required in both the baseline and study end data extracts. Extensive data cleaning and matching was conducted prior to analysis (Supporting Information, 3. Hierarchic matching strategy).
Outcomes
The primary outcome was the proportion of patients with high CVD risk who were not receiving preventive medications at baseline (ie, undertreated patients with high CVD risk) who achieved both blood pressure (≤ 140/90 mmHg; ≤ 130/80 mmHg in people with diabetes or albuminuria) and LDL cholesterol goals (< 2.0 mmol/L) by study end. High CVD risk was defined as a history of established CVD, having high risk conditions (diabetes in people over 60 years of age, diabetes and albuminuria, stage 3B or worse chronic kidney disease, systolic blood pressure ≥ 180 mmHg, diastolic blood pressure ≥ 110 mmHg, or total cholesterol level > 7.5 mmol/L), or a calculated 5‐year CVD risk exceeding 15%, as assessed with the 1991 Framingham equation.23 Full preventive treatment was defined as comprising at least one blood pressure‐lowering medication and a statin (as recommended by Australian National Vascular Disease Prevention Alliance guidelines17), as well as an antiplatelet medication for patients with established athero‐thrombotic CVD.
Secondary outcomes were:
- proportions of undertreated patients with high CVD risk who achieved either blood pressure or LDL cholesterol targets, or for whom treatment was intensified (newly prescribed or additional antiplatelet, blood pressure‐lowering, or lipid‐lowering drugs);
- mean changes in systolic and diastolic blood pressure and lipid levels for undertreated patients at high CVD risk;
- proportion of all patients with high baseline CVD risk who achieved both blood pressure and LDL cholesterol targets and were prescribed antiplatelet medication (if diagnosed with CVD);
- proportion of patients without high baseline CVD risk who received a new prescription or intensified prescribing of blood pressure‐ or lipid‐lowering or antiplatelet medications;
- proportion of all patients aged 45 years or more (35 years for Aboriginal and Torres Strait Islander Australians) with appropriate CVD risk factor screening: recorded smoking status and systolic blood pressure at least once during the preceding 12 months, and total and high‐density lipoprotein cholesterol levels recorded at least once during the preceding 24 months.
Statistical analysis
Statistical analyses were performed in SAS Enterprise Guide 7.15. To detect a relative risk (intervention v control) of at least 1.35 in the proportion of patients achieving blood pressure and LDL cholesterol targets, we estimated that 70 practices (35 per study arm), with a mean cluster size of 60, were required to provide 80% power (α = 0.05, two‐tailed). We assumed an intra‐class correlation of 0.01, and that 10% of control group patients would achieve the primary study outcome. Data analyses were performed on an intention‐to‐treat basis. The primary outcome and binary measure outcomes were assessed in hierarchic log‐binomial regression models (with practice as a random effect). Continuous outcomes were analysed in mixed models with an unstructured correlation structure. Complete case analyses are reported, as are two sensitivity analyses applying different methods for handling missing data. Pre‐defined subgroup analyses were performed, including by service size, age, sex, type of high risk, and diabetes status (further details: Supporting Information, 4. Statistical analysis).
Ethics approval
The protocol for this investigation was approved by the human research ethics committees of the University of Sydney (reference, 2015/616), the University of Notre Dame Australia (reference, 016011S), Monash University (reference, CF16/780‐2016000378), and Curtin University (reference, HRE2017‐0157). As patient data were obtained from de‐identified EMR extracts, written informed consent by patients was waived, except for participants prescribed polypills and those enrolled in the pharmacy adherence program.
Results
A total of 71 general practices were recruited between 14 December 2016 and 14 August 2018 (Box 1) and participated for a minimum of 12 months (median, 15 months; interquartile range, 14–20 months). One control group practice closed during the study and was excluded because it provided no follow‐up data. Practices were located in four Australian states; 29 of the 70 belonged to one corporate operator (MyHealth). Fourteen of the 36 partner pharmacies belonged to national chains, 15 were independently owned, and seven were franchises (Supporting Information, table 2).
Study cohorts
Baseline and end‐of‐study data were available for 74 608 eligible patients; 13 264 were deemed to be at high CVD risk at baseline, of whom 7165 were undertreated (the primary outcome cohort) (Box 1). The mean age of patients in the primary outcome cohort at baseline was 67.9 years (standard deviation [SD], 14.0 years), and 3146 were women (44%); 2849 had diabetes (40%) and 2631 established CVD (37%). Their mean systolic blood pressure was 134.4 mmHg (SD, 18.6 mmHg), mean LDL cholesterol level 3.0 mmol/L (SD, 1.2 mmol/L), and mean body mass index (BMI) 29.3 kg/m2 (SD, 6.3 kg/m2). These characteristics were similar in the intervention and control groups, as were those for all participants with high CVD risk at baseline and for all participants (Box 2; Supporting Information, table 3).
Intervention uptake
In the intervention group, HealthTracker was used at least once for 347 of 3236 undertreated patients with high baseline CVD risk (10.7%), for 754 of 6084 patients with high CVD risk (12.4%), and for 2662 of all 35 124 patients (7.6%) (Box 3). Polypills were prescribed and dispensed to 107 of 6757 patients with high baseline CVD risk (1.6%), without clear preference by drug combination (Supporting Information, table 1); these patients attended 20 of the 35 intervention practices. A total of 36 patients participated in the ARMS‐7 screening phase of the PASS program, of whom 18 scored greater than 7 and consented to the full program. Median duration of support provided to intervention practices by the study team was 11.2 hours (interquartile range, 8.3–14 h; range, 5.4–27 h).
Intervention effectiveness
Follow‐up blood pressure and LDL cholesterol (primary outcome) data were available for 4477 patients in the primary outcome cohort (62%: 2156 intervention, 2321 control patients); the baseline characteristics of those with and without missing follow‐up primary outcome data were similar (Supporting Information, table 4). At study end, 423 of 2156 undertreated patients with high baseline CVD risk in intervention practices (19.6%) and 466 of 2321 in control practices (20.1%) had achieved their blood pressure and LDL‐cholesterol targets (relative risk [RR], 1.06; 95% CI, 0.85–1.32) (Box 4).
Differences in secondary outcomes between the control and intervention groups were not statistically significant, including the increased prescribing of any guideline‐recommended medications for undertreated patients with high CVD risk (intervention: 877 of 3588 patients, 24.4%; control: 959 of 3577 patients, 26.8%; RR, 0.94; 95% CI, 0.76–1.16) and appropriate CVD risk factor screening for all patients aged 45 years or more at baseline, or 35 years or more for Indigenous Australians (intervention: 12 837 of 21 894 patients, 58.6%; control: 13 319 of 21 425 patients, 62.2%; RR, 0.99; 95% CI, 0.82–1.20) (Box 4; Supporting Information, table 5). Service size, patient sex and age, having diabetes, and type of CVD high risk did not influence intervention effectiveness (Box 5).
The results of sensitivity analyses adjusted for missing data were similar to those of the main analysis (Supporting Information, table 6). A post hoc analysis did not identify any association between HealthTracker use and the primary outcome, regardless of adjustment for missing data (Supporting Information, table 7).
Discussion
In the INTEGRATE study, our primary health care intervention for managing CVD risk factors was not fully adopted by the participating general practices and pharmacies, and it did not improve CVD risk factor assessment or increase the prescribing of recommended medications. The HealthTracker decision support tool was used for only 10.7% of patients in the INTEGRATE primary outcome cohort, so that the opportunity for improving their management was very limited. Polypills were prescribed for fewer than 2% of eligible patients, and PASS was used even less frequently.
The findings of a mixed methods process evaluation of the intervention will be published separately. We suspect that that the complexity of the intervention, difficulties in integrating the intervention into the usual clinical workflow, uncertainty about the future availability of polypills, and inadequate incentives for quality improvement in general practice reimbursement models may have inhibited its uptake. Central support for the intervention primarily involved overcoming user problems with the intervention technology, including password retrievals when participants forgot their login details. PASS was incompatible with some pharmacy software systems, and this probably contributed to its extremely limited use. To ensure its usability, HealthTracker was deployed within a third party program, and users needed to add a step to their usual workflow to obtain recommendations. Further, we could not obtain an informed consent waiver for prescribing polypills, and obtaining consent also disturbed the normal clinical workflow. After the initial payment for setting up the intervention, there were no financial incentives for promoting its components. Health care provider income in Australia is largely based upon volume and fees for specific services. While value‐based systems and reimbursement mechanisms have been proposed, their implementation largely remains exploratory.24,25
Strengths and limitations
Strengths of the INTEGRATE trial included its prospective randomised design, the analysis of routinely collected data to maximise sample size and efficiency, and the assessment of outcomes on the basis of data that doctors use for treatment decisions. Including a broader range of general practices than similar studies10,11 was important for assessing the implementation of the intervention.
The considerable proportion of missing follow‐up data, the consequence of using EMR data to assess outcomes, was a limitation. However, the problem was similar in degree for the intervention and control groups, and thus unlikely to have introduced systematic bias, a view confirmed by the results of our sensitivity analyses.
Conclusion
The multifaceted INTEGRATE intervention was not broadly implemented and did not improve CVD risk management in participating Australian general practices. The complexity of the intervention, despite evidence for the efficacy of its individual components, may have inhibited its adoption. A detailed process evaluation will provide greater insight into this problem, as well as into the influence of broader contextual factors, such as the infrastructure, policy, and reimbursement environment in which any quality improvement initiative must operate.
Box 1 – Flow diagram of the parallel arm, cluster randomised trial of INTEGRATE, an electronic decision support‐based intervention for improving management of cardiovascular risk in primary health care
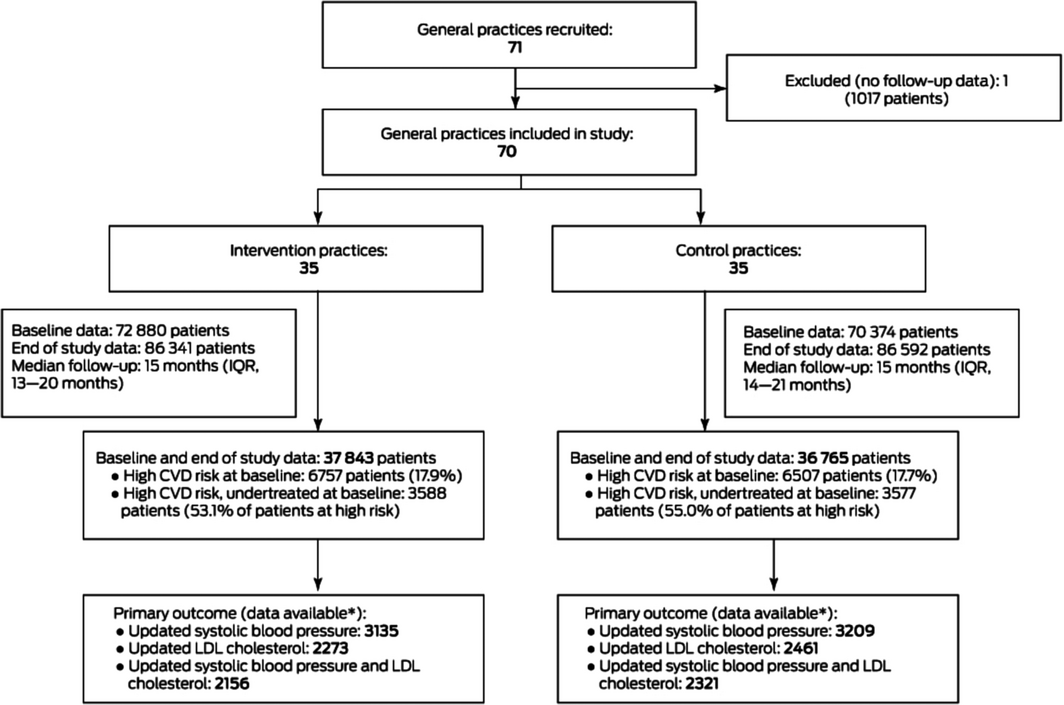
IQR = interquartile range; LDL = low density lipoprotein. * Patients for whom systolic blood pressure and LDL cholesterol data were available, with at least one updated value for each parameter since baseline.
Box 2 – Baseline characteristics of the participants in the INTEGRATE trial, by analysis cohort*
Characteristic |
All participants |
Participants at high CVD risk (baseline) |
Participants at high CVD risk and undertreated (baseline) |
||||||||||||
Intervention |
Control |
Intervention |
Control |
Intervention |
Control |
||||||||||
|
|||||||||||||||
Number of patients |
37 843 |
36 765 |
6757 |
6507 |
3588 |
3577 |
|||||||||
Age (years), mean (SD) |
50.4 (18.4) |
50.7 (18.3) |
69.3 (12.5) |
69.3 (12.6) |
67.9 (14.0) |
67.8 (14.0) |
|||||||||
Sex (women) |
22 228 (58.7%) |
21 787 (59.3%) |
2883 (42.7%) |
2776 (42.7%) |
1588 (44.3%) |
1558 (43.6%) |
|||||||||
Current smoker or quit in past 12 months |
4547/33 743 (13.5%) |
4442/32 975 (13.5%) |
963/6178 (15.6%) |
889/6025 (14.8%) |
582/3278 (17.8%) |
553/3304 (16.7%) |
|||||||||
Diabetes |
4333 (11.4%) |
4146 (11.3%) |
3294 (48.7%) |
3219 (49.5%) |
1413 (39.4%) |
1436 (40.1%) |
|||||||||
Body mass index (kg/m2), mean (SD) |
28.8 (6.6) |
28.4 (6.4) |
30.1 (6.49) |
29.7 (6.10) |
29.6 (6.6) |
29.0 (6.1) |
|||||||||
Systolic blood pressure (mmHg), mean (SD) |
125.3 (16.8) |
125.7 (16.3) |
134.1 (18.3) |
134.1 (17.6) |
134.3 (19.0) |
134.6 (18.3) |
|||||||||
Diastolic blood pressure (mmHg), mean (SD) |
77.4 (10.7) |
77.8 (10.3) |
77.1 (12.05) |
77.3 (11.5) |
78.4 (12.5) |
78.8 (11.8) |
|||||||||
Achieved blood pressure target |
NA |
NA |
4379/6528 |
4218/6227 |
2264/3437 |
2201/3364 |
|||||||||
Total cholesterol (mmol/L), mean (SD) |
5.0 (1.1) |
5.0 (1.1) |
4.7 (1.4) |
4.7 (1.4) |
5.2 (1.5) |
5.2 (1.5) |
|||||||||
HDL cholesterol (mmol/L), mean (SD) |
1.4 (0.4) |
1.4 (0.4) |
1.3 (0.38) |
1.3 (0.41) |
1.3 (0.4) |
1.3 (0.4) |
|||||||||
LDL cholesterol (mmol/L), mean (SD) |
2.9 (0.9) |
2.9 (1.0) |
2.6 (1.2) |
2.6 (1.2) |
3.0 (1.2) |
3.0 (1.2) |
|||||||||
Achieved LDL cholesterol target |
NA |
NA |
2041/6028 |
1981/5822 |
599/3097 |
593/3090 (19.2%) |
|||||||||
Achieving blood pressure or LDL cholesterol target |
NA |
NA |
4933/6757 |
4777/6507 |
2431/3588 |
2383/3577 (66.6%) |
|||||||||
Achieved blood pressure and LDL cholesterol targets |
NA |
NA |
1487/5897 |
1422/5650 |
432/3021 |
411/2959 (13.9%) |
|||||||||
Triglycerides (mmol/L), mean (SD) |
1.5 (1.0) |
1.5 (1.0) |
1.8 (1.3) |
1.8 (1.2) |
1.8 (1.4) |
1.7 (1.1) |
|||||||||
Creatinine (µmol/L), mean (SD) |
75.4 (30.8) |
74.4 (27.9) |
90.4 (57.2) |
88.7 (51.4) |
89.2 (60.8) |
87.5 (56.5) |
|||||||||
Unable to calculate CVD risk |
15 180 (40.1%) |
14 129 (38.4%) |
NA |
NA |
NA |
NA |
|||||||||
High CVD risk |
6757 (17.9%) |
6507 (17.7%) |
6757 (100%) |
6507 (100%) |
3588 (100%) |
3577 (100%) |
|||||||||
History of CVD |
2729 (7.2%) |
2613 (7.1%) |
2729 (40.4%) |
2613 (40.2%) |
1306 (36.4%) |
1325 (37.0%) |
|||||||||
High risk condition |
3335 (8.8%) |
3264 (8.9%) |
3335 (49.4%) |
3264 (50.2%) |
1745 (48.6%) |
1749 (48.9%) |
|||||||||
High calculated risk |
693 (1.8%) |
630 (1.7%) |
693 (10.3%) |
630 (9.7%) |
537 (15.0%) |
503 (14.1%) |
|||||||||
High‐risk and undertreated† |
NA |
NA |
3588 (53.1%) |
3577 (55.0%) |
3588 (100%) |
3577 (100%) |
|||||||||
|
|||||||||||||||
CVD = cardiovascular disease; HDL = high‐density lipoprotein; LDL = low‐density lipoprotein; NA = not applicable; SD = standard deviation. * The overall numbers are for all patients who had records that could be matched at baseline and end of the study for a category. Numbers of patients are indicated when data for that category were not available at baseline. † Not prescribed blood pressure‐lowering drugs, statin, or (for people with history of CVD) antiplatelet medication. |
Box 3 – Implementation of the intervention components, by component and patient group
Intervention component |
Practices that used component for at least one patient |
Patients for whom component was used |
|||||||||||||
At least once |
Number per site, median (IQR) |
Mean site proportion (SD) |
|||||||||||||
|
|||||||||||||||
HealthTracker* |
|
|
|
|
|||||||||||
All patients (35 124 patients) |
32/34 (94%) |
2662 (7.6%) |
5.1 (3.2–10) |
9.9% (12.4%) |
|||||||||||
Patients with high CVD risk at baseline (6084 patients) |
31/34 (91%) |
754 (12.4%) |
9.0 (4.6–20) |
17.1% (19.9%) |
|||||||||||
Patients with high CVD risk and undertreated at baseline (3236 patients) |
30/34 (88%) |
347 (10.7%) |
7.5 (4.8–15) |
15.1% (18.0%) |
|||||||||||
Polypills |
|
|
|
|
|||||||||||
Patients with high CVD risk at baseline (6757 patients) |
20/35 (57%) |
107 (1.6%) |
NA |
NA |
|||||||||||
Pharmacy Adherence Support Service (PASS)† |
|
|
|
|
|||||||||||
All patients |
15/36 (42%) |
36‡ |
NA |
NA |
|||||||||||
|
|||||||||||||||
CVD = cardiovascular disease; IQR = interquartile range; NA = not applicable; SD = standard deviation. * One site excluded because of technical problems prevented access to HealthTracker data. † The PASS program was available at 36 pharmacies. One GP practice had two locations but a joint database and was classified as one site, but each location had a partner pharmacy (ie, two pharmacies linked to one GP site). ‡ 36 patients were assessed with the Adherence to Refills and Medications Scale (ARMS‐7), of whom 18 scored greater than 7 and consented to participate in the full PASS program. |
Box 4 – Outcomes for participants in the INTEGRATE trial, by analysis cohort
Outcome |
Intervention |
Control |
Outcome: intervention v control (95% CI) |
Intra‐class correlation coefficient |
|||||||||||
|
|||||||||||||||
Patients at high CVD risk and undertreated at baseline |
3588 |
3577 |
|
|
|||||||||||
Patients with baseline and follow‐up data for primary outcome |
2156 (60.1%) |
2321 (64.9%) |
|
|
|||||||||||
Achieved blood pressure and LDL cholesterol targets (primary outcome) |
423/2156 (19.6%) |
466/2321 (20.1%) |
RR, 1.06 (0.85–1.32) |
0.03 |
|||||||||||
Achieved blood pressure targets |
2185/3135 (69.7%) |
2153/3209 (67.1%) |
1.05 (0.98–1.13) |
0.03 |
|||||||||||
Achieved LDL cholesterol target |
599/2273 (26.4%) |
650/2461 (26.4%) |
1.06 (0.88–1.27) |
0.03 |
|||||||||||
Achieved blood pressure or LDL cholesterol targets |
1684/2156 (78.1%) |
1730/2321 (74.5%) |
1.05 (0.99–1.12) |
0.04 |
|||||||||||
Escalation of any guideline‐recommended medication prescribing |
877 (24.4%) |
959 (26.8%) |
RR, 0.94 (0.76–1.16) |
0.06 |
|||||||||||
Escalation of blood pressure‐lowering drugs |
496 (13.8%) |
636 (17.8%) |
RR, 0.83 (0.64–1.09) |
0.07 |
|||||||||||
Escalation of lipid‐lowering drugs |
378 (10.5%) |
429 (12.0%) |
RR, 1.05 (0.78–1.43) |
0.10 |
|||||||||||
Escalation of antiplatelet drugs |
184/3588 (5.1%) |
247/3577 (6.9%) |
RR, 0.77 (0.56–1.07) |
0.04 |
|||||||||||
All patients with high baseline CVD risk |
6757 |
6507 |
|
|
|||||||||||
Achieved blood pressure and LDL‐cholesterol targets |
1420 (21.0%) |
1368 (21.0%) |
RR, 1.02 (0.84–1.24) |
0.02 |
|||||||||||
Screening population * |
21 894 |
21 425 |
|
|
|||||||||||
Received appropriate screening |
12 837 (58.6%) |
13 319 (62.2%) |
RR, 0.99 (0.82–1.20) |
0.11 |
|||||||||||
|
|||||||||||||||
CI = confidence interval; CVD = cardiovascular disease; LDL = low‐density lipoprotein; MD = mean difference; RR = relative risk. * Patients aged 45 years or more at baseline, or 35 years or more for Aboriginal and Torres Strait Islander Australians. Appropriate screening includes having smoking status and systolic blood pressure recorded at least once during the preceding 12 months, and total cholesterol and high‐density lipoprotein cholesterol during the preceding 24 months. |
Received 22 June 2020, accepted 13 November 2020
Abstract
Objectives: To determine whether a multifaceted primary health care intervention better controlled cardiovascular disease (CVD) risk factors in patients with high risk of CVD than usual care.
Design, setting: Parallel arm, cluster randomised trial in 71 Australian general practices, 5 December 2016 – 13 September 2019.
Participants: General practices that predominantly used an electronic medical record system compatible with the HealthTracker electronic decision support tool, and willing to implement all components of the INTEGRATE intervention.
Intervention: Electronic point‐of‐care decision support for general practices; combination cardiovascular medications (polypills); and a pharmacy‐based medication adherence program.
Main outcome measures: Proportion of patients with high CVD risk not on an optimal preventive medication regimen at baseline who had achieved both blood pressure and low‐density lipoprotein (LDL) cholesterol goals at study end.
Results: After a median 15 months’ follow‐up, primary outcome data were available for 4477 of 7165 patients in the primary outcome cohort (62%). The proportion of patients who achieved both treatment targets was similar in the intervention (423 of 2156; 19.6%) and control groups (466 of 2321; 20.1%; relative risk, 1.06; 95% CI, 0.85–1.32). Further, no statistically significant differences were found for a number of secondary outcomes, including risk factor screening, preventive medication prescribing, and risk factor levels. Use of intervention components was low; it was highest for HealthTracker, used at least once for 347 of 3236 undertreated patients with high CVD risk (10.7%).
Conclusions: Despite evidence for the efficacy of its individual components, the INTEGRATE intervention was not broadly implemented and did not improve CVD risk management in participating Australian general practices.
Trial registration: Australian New Zealand Clinical Trials Registry, ACTRN12616000233426 (prospective).