Accurate interpretation of electrocardiographs (ECGs) is essential for doctors to avoid diagnostic errors and delays in time-critical management. Diagnostic errors can result from knowledge deficits but also from cognitive biases and heuristics (mental shortcuts).1-4 Clinical reasoning consists of two distinct, although not exclusive, types of mental processes — analytical and non-analytical.1 In emergency departments (EDs), doctors are often required to interpret results of diagnostic tests such as ECGs with limited or evolving clinical information, and adapt by using non-analytical clinical reasoning strategies such as pattern recognition and heuristics.4 Heuristics have been associated with enhanced diagnostic accuracy,5 but may also predispose to diagnostic error caused by bias.4,5 The major cognitive biases in medicine are anchoring bias (tendency to fixate on first impressions), confirmation bias (seeking features to confirm rather than refute a diagnosis), and premature closure (accepting a diagnosis before it has been fully verified).4
Access to clinical history can both enhance diagnostic accuracy and increase diagnostic errors.6-11 A systematic review of the interpretation of radiology and pathology test results has shown that adding clinical history is associated with improved diagnostic accuracy.6 Some studies of ECG interpretation have shown that providing a clinical history or vignette has no significant effect on diagnostic accuracy,10,11 but others have shown that history suggestive of the correct diagnosis increases diagnostic accuracy and misleading history decreases diagnostic accuracy.7-9
ECGs were obtained from hospital patients, textbooks and internet-based sources. They were reviewed without clinical history by a cardiologist and a senior emergency physician, and were included in the study only if these doctors agreed on the diagnosis. Thirty ECGs representing 10 clinically important diagnoses were included (three ECGs per diagnosis). These diagnoses are shown in Box 1.
The 30 ECGs were randomly divided into three packs of 10, such that there was one example of each diagnosis per pack. Each ECG was preceded by positively biased, negatively biased, or no clinical history. Positively biased history was suggestive of the correct diagnosis and negatively biased history was suggestive of an alternative diagnosis (Box 1). A random number generator was used to order the ECGs within each pack and assign a different category of history to each ECG.
This was an exploratory study and an a priori sample size calculation was not undertaken. An estimate of the required sample size was obtained from previous studies that investigated similar outcomes.7-12 A difference in mean scores of 1 out of 10 (ie, 10% difference) between clinical history categories was considered clinically important. Assuming a correlation coefficient of 0.50 between repeated observations on the same participant, a total group size of 129 would provide 80% power at 0.025 alpha level (significance level adjusted to account for multiple pairwise comparisons) to detect a difference in mean scores of 0.55.
The mean accuracy of ECG interpretation for all participants was 52% (95% CI, 50%–53%). For junior doctors, mean accuracy was 42% (95% CI, 40%–44%); for senior doctors, it was 65% (95% CI, 62%–67%). Participants were most accurate for the ECG diagnoses of ST elevation myocardial infarction and ventricular tachycardia and least accurate for Brugada pattern and long QT interval. The percentages of ECGs that were correctly interpreted for each diagnosis are shown in Box 2, and these data are further divided by clinical history in Box 3.
In multivariable analysis, there was no evidence of significant effect modification between clinical history and participant seniority (interaction term P = 0.21). As a result, the interaction term was removed and only the main effects of history and seniority were included in the final model. There was a significant association between the category of history provided and the accuracy of ECG interpretation. Both mean and median test scores were significantly higher when the ECG was presented with positively biased history compared with no history, and lower when the ECG was presented with negatively biased history (Box 4).
In the final multivariable GEE model, the predicted mean score for senior doctors provided with no clinical history was 6.25 (95% CI, 5.90–6.62). Positively biased history was associated with a 42% (95% CI, 35%–49%) increase in mean test scores compared with no history. Negatively biased history was associated with a 34% (95% CI, 29%–39%) decrease in mean test scores compared with no history. Junior doctors’ mean test scores were 34% (95% CI, 29%–40%) lower than those of senior doctors (Box 5). These effects are adjusted for other predictors in the model.
Our study shows that introduction of bias into clinical history significantly affects diagnostic accuracy of ECG interpretation. On one hand, doctors were more accurate when provided with history suggestive of the correct ECG diagnosis. The history may have directed participants to search for specific ECG findings or alerted them to possible diagnoses that they might not have otherwise considered. Clinicians may have also combined history with ECG findings to make their ECG diagnoses, rather than interpreting ECGs independently.13 This suggests that ECG interpretation may not be independent of the pre-test probability, contrary to the traditional Bayesian approach to clinical reasoning.
On the other hand, clinical history suggestive of an alternative diagnosis had a detrimental effect on diagnostic accuracy. Cognitive processes such as anchoring bias, confirmation bias and premature closure may have contributed to participants ignoring findings consistent with the correct ECG diagnoses. This is consistent with results of research which show that diagnostic suggestion can lead to interpretation errors, particularly in the context of atypical or inconsistent clinical histories.14-16 Clinicians therefore need to ensure that any history obtained before the interpretation of ECGs is reliable and accurate. Furthermore, strategies are required so that an incorrect or atypical history does not adversely affect the interpretation of diagnostic test results.
Several such strategies have been proposed to reduce the risk of diagnostic error.1-4,9,13-18 One approach is to interpret test results without clinical history (to facilitate unbiased reading) and then reinterpret with the history.14 Another approach is to seek more clinical information when ECG findings are inconsistent with history. A “combined reasoning approach” — the deliberate use of both analytical and non-analytical (intuitive) methods — has been associated with increased diagnostic accuracy for ECG interpretation.17 Another strategy is for clinicians to develop an understanding of basic error theory and skills in meta-cognition (ie, think about their thinking18), and use specific strategies to counter cognitive pitfalls.4 Despite multiple strategies being proposed, there is little evidence to support one approach over another.18
The strengths of this study include the blinded assessment of the ECG responses to minimise misclassification bias, the good inter-rater agreement, and the randomisation of ECGs within each pack to minimise the effect of learning bias. In contrast to previous similar studies,7-11 this was a multicentre study with a specific focus on the effect of clinical history and cognitive bias in the ED setting.
However, there were a number of limitations. There were not enough participants to enable subgroup analyses of each clinical designation. We did not control for other potential confounders such as hospital type or prior ECG training. Positively and negatively biased clinical histories provided were formulated by the authors and may have caused a bias in favour of the observed differences. Although the ECGs in our study were included only if both the emergency physician and cardiologist agreed on the diagnosis, significant inter-observer variability in ECG interpretation often exists even among cardiologists (who are frequently the “gold standard” reference in similar studies).19 The accuracy of ECG interpretation observed in this study was probably lower than in clinical practice as a result of the strict marking scheme, designed to avoid ambiguity and marker bias. Some of the participants whose answers were marked as incorrect might have identified features in the ECGs that would have led to further enquiry in clinical practice. The clinical significance of these interpretation errors is unknown.
The decreased accuracy of ECG interpretation associated with negatively biased clinical history was consistent across senior and junior levels. In contrast, another study has shown that expertise can mitigate the detrimental effect of bias — providing a misleading history decreased diagnostic accuracy of ECG interpretation by 5% for cardiologists but up to 25% for residents.7 In our study, no such protective effect of expertise was seen, possibly because most of the senior doctors were emergency registrars and few were emergency physicians.
Overall accuracy of ECG interpretation in this study was 52%, which is comparable to findings of previous studies — accuracies of 36%–96% for emergency doctors.19 The performance of senior doctors was comparable to previously reported accuracies of 53%–96% for cardiologists.7,19 Accuracies for the individual ECG diagnoses in our study were similar to those reported in a recent Australian study.12 The low diagnostic accuracy for some ECG patterns suggests that knowledge deficits may have been just as important as cognitive bias in contributing to diagnostic error. This is supported by the finding that the effect of seniority was similar to that of biased clinical history on the accuracy of ECG interpretation. Junior doctors may have been unable to identify ECG patterns of Brugada pattern and long QT interval due to a lack of prior exposure to these disorders. Knowledge gaps are more amenable to educational interventions and highlight that ongoing training in ECG interpretation is an important component of postgraduate education programs.
1 Clinical histories provided for each electrocardiograph (ECG) diagnosis
3 Percentages of electrocardiographs (ECGs) that were correctly interpreted, by ECG diagnosis and clinical history
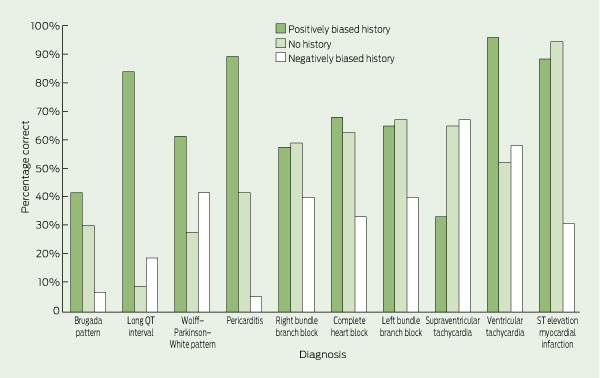
Received 15 December 2011, accepted 2 July 2012
- Monique F Cruz1
- James Edwards1
- Michael M Dinh1
- Elizabeth H Barnes2
- 1 Emergency Department, Royal Prince Alfred Hospital, Sydney, NSW.
- 2 NHMRC Clinical Trials Centre, University of Sydney, Sydney, NSW.
We thank Raj Puranik (cardiologist) and Tim Royle (senior emergency physician) for reviewing the ECGs included in the study, and Samantha Bendall and Rosslyn Hing (emergency physicians) for marking the ECG answers.
Michael Dinh was awarded a grant by the New South Wales Government Health Education and Training Institute to develop and implement an online ECG training and knowledge-sharing platform.
- 1. Norman GR, Eva KW. Diagnostic error and clinical reasoning. Med Educ 2010; 44: 94-100.
- 2. Berner ES, Graber ML. Overconfidence as a cause of diagnostic error in medicine. Am J Med 2008; 121 (5 Suppl): S2-S23.
- 3. Graber ML. Educational strategies to reduce diagnostic error: can you teach this stuff? Adv Health Sci Educ Theory Pract 2009; 14 Suppl 1: 63-69.
- 4. Croskerry P. The importance of cognitive errors in diagnosis and strategies to minimize them. Acad Med 2003; 78: 775-780.
- 5. Sibbald M, Cavalcanti RB. The biasing effect of clinical history on physical examination diagnostic accuracy. Med Educ 2011; 45: 827-834.
- 6. Loy CT, Irwig L. Accuracy of diagnostic tests read with and without clinical information: a systematic review. JAMA 2004; 292: 1602-1609.
- 7. Hatala R, Norman GR, Brooks LR. Impact of a clinical scenario on accuracy of electrocardiogram interpretation. J Gen Intern Med 1999; 14: 126-129.
- 8. Hatala RA, Norman GR, Brooks LR. The effect of clinical history on physician’s ECG interpretation skills. Acad Med 1996; 71 (10 Suppl): S68-S70.
- 9. LeBlanc VR, Dore K, Norman GR, Brooks LR. Limiting the playing field: does restricting the number of possible diagnoses reduce errors due to diagnosis-specific feature identification? Med Educ 2004; 38: 17-24.
- 10. Grum CM, Gruppen LD, Wolliscroft JO. The influence of vignettes on EKG interpretation by third-year students. Acad Med 1993; 68 (10 Suppl): S61-S63.
- 11. Dunn PM, Levinson W. The lack of effect of clinical information on electrocardiographic diagnosis of acute myocardial infarction. Arch Intern Med 1990; 150: 1917-1919.
- 12. Hoyle RJ, Walker KJ, Thomson G, Bailey M. Accuracy of electrocardiogram interpretation improves with emergency medicine training. Emerg Med Australas 2007; 19: 143-150.
- 13. Irwig L, Macaskill P, Walter SD, Houssami N. New methods give better estimates of changes in diagnostic accuracy when prior information is provided. J Clin Epidemiol 2006; 59: 299-307.
- 14. Griscom NT. A suggestion: look at the images first, before you read the history. Radiology 2002; 223: 9-10.
- 15. Leblanc VR, Norman GR, Brooks LR. Effect of a diagnostic suggestion on diagnostic accuracy and identification of clinical features. Acad Med 2001; 76 (10 Suppl): S18-S2.
- 16. Leblanc VR, Brooks LR, Norman GR. Believing is seeing: the influence of a diagnostic hypothesis on the interpretation of clinical features. Acad Med 2002; 77 (10 Suppl): S67-S69.
- 17. Eva KW, Hatala RM, Leblanc VR, Brooks LR. Teaching from the clinical reasoning literature: combined reasoning strategies help novice diagnosticians overcome misleading information. Med Educ 2007; 41: 1152-1158.
- 18. Scott IA. Errors in clinical reasoning: causes and remedial strategies. BMJ 2009; 338: b1860.
- 19. Salerno SM, Alguire PC, Waxman HS. Competency in interpretation of 12-lead electrocardiograms: a summary and appraisal of published evidence. Ann Intern Med 2003; 138: 751-760.
Abstract
Objective: To investigate whether bias in clinical history affects accuracy of electrocardiograph (ECG) interpretation among doctors working in emergency departments.
Design and setting: Observational study conducted at four teaching hospitals in Sydney from May to September 2011.
Participants: Emergency registrars and physicians (senior doctors), and interns, resident medical officers and senior resident medical officers (junior doctors).
Intervention: Participants interpreted 30 ECGs representing 10 diagnoses. ECGs were provided with positively biased history (suggestive of the correct diagnosis), negatively biased history (suggestive of an alternative diagnosis) or no history.
Main outcome measures: Accuracy of ECG interpretation, measured as a score out of 10 (for each category of clinical history) and as a percentage of correctly interpreted ECGs.
Results: Of 307 doctors who were sent a recruitment email for the study, 132 participated (43%). The overall mean accuracy of ECG interpretation was 52% (95% CI, 50%–53%). For junior doctors, mean accuracy was 42% (95% CI, 40%–44%); for senior doctors, it was 65% (95% CI, 62%–67%). In adjusted models, the mean predicted score for senior doctors provided with no history was 6.25 (95% CI, 5.90–6.62) with junior doctors obtaining mean scores 34% lower than senior doctors (95% CI, 29%–40%; P < 0.001). Compared with no history, positively biased history was associated with 42% higher mean scores (95% CI, 35%–49%; P < 0.001) and negatively biased history was associated with 34% lower mean scores (95% CI, 29%–39%; P < 0.001).
Conclusion: Bias in clinical history significantly influenced the accuracy of ECG interpretation. Strategies that reduce the detrimental impact of cognitive bias and improved ECG training for doctors are recommended.